DATA GOVERNANCE: THE QUEST FOR QUALITY
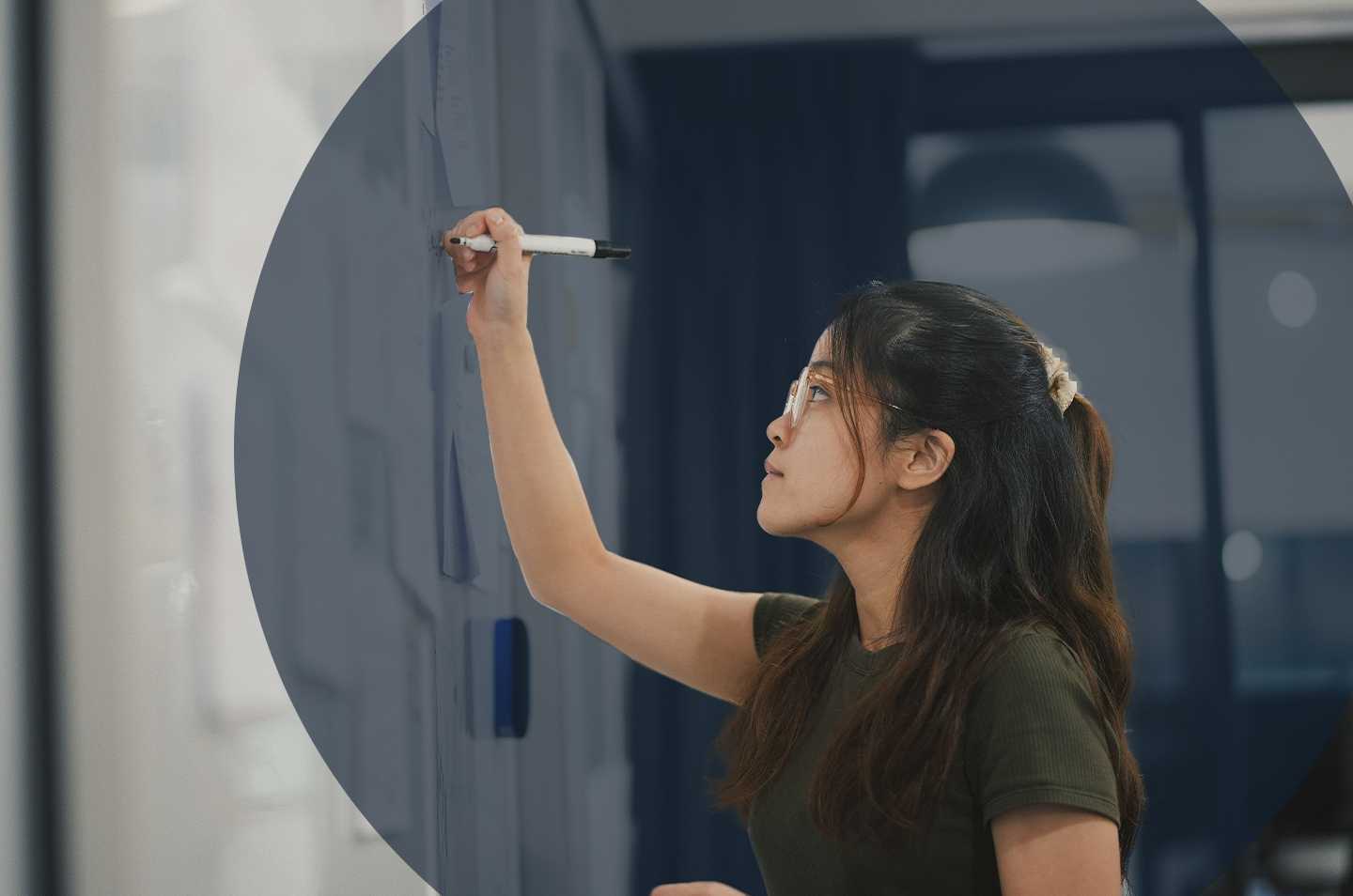
We are living in a world where the importance of data is growing exponentially, but the ability to track, store, and manage it has become a major undertaking. This is a formidable challenge for virtually every industry, as organizations have to not only manage the volume and complexity of data, but also adhere to complex regulatory mandates. With stringent guidelines put in place by regulatory authorities, these comprehensive information sources must be rigorously maintained and reported. And while many larger organizations are somewhat able to manage, mid-size and smaller firms are falling behind in their ability to transform at the required rate.
The Root Causes
One of the primary reasons for this problem is simply organic growth that introduces new and expanding swaths of data, but other conditions which could be stalling companies’ ability to maintain data quality include frequent M&A and siloed business operations. Another aspect of the problem is legacy systems that are unable to take advantage of these modern-day data governance and quality tools. No matter the cause, the results are typically the same: heightened maintenance costs, poor data quality/context, and the increasing inability to comply with regulations.
What these organizations need is a sustainable data governance and quality program – defined as the disciplined management of processes to acquire, consolidate, validate, and enrich critical data elements. Such a program has the potential to help enterprises improve their ability to manage and standardize information as well as to provide consistency to align with regulatory authorities.
The Cost of Poor Data Quality
Poor data quality is an invisible drag on enterprises which is too often embedded in their culture and seen simply as the cost of doing business. Recent penalties—some of which cost organizations upwards of $1B—make this abundantly clear. Larger firms may be able to shoulder these penalties, but for midsize and smaller firms, inaction is not an option.
Defining Critical Data Elements
First and foremost, it is vital to develop an understanding of ‘critical data elements.’ These critical data elements are comprised of the fundamental details on customers, accounts, security, and transactions – not the operational information required to perform end-to-end transactions. In other words, the critical data elements include static information that identifies and qualifies the transaction. Overall, they constitute about half of the information involved in every transaction and include:
- Customer & Counterparty information: Descriptions of legal entities, including the identification, contact, and account data as well as information on relationships with family members, attorneys, accountants, etc.
- Securities: Basic security information, terms, conditions, pricing, customer market value reporting, etc.
Example: Banking & Capital Markets
To demonstrate the importance of well-defined critical data elements, let’s explore an example within an industry that is uniquely dependent on high-quality data: the Banking and Capital Markets industry.
These critical data elements are integral in ensuring that bankers are complying with regulatory mandates, traders are trading what they are expected to trade, and that customer statements show the correct information at the right market valuation. However, these data elements and this overall concept is frequently given very little priority. What’s more, there is currently no uniform standard for the classification, management, and quality of these data sets. As a result, the industry is suffering from a lack of data integrity, disjointed transactions, and fragmented business processes and technologies.
Breaking the Bank
Why are standardized critical data elements so important for banking and capital markets? Put simply, poor quality reference data represents enormous costs for this industry in particular. These outsized costs include:
- Excessive maintenance expenses
- Duplication & redundancy across product siloes
- Failed trade
- Fines & penalties
- High transaction costs
Prevalent Obstacles
It’s not that banking and capital market firms are unaware of these issues. In fact, most have funds allocated for these initiatives, but challenges are driven by low prioritization and limited executive buy-in. As a result, these organizations suffer from a lack of long-term strategic and technological commitment, scarce resources, and difficulty quantifying ROI.
Final Thoughts
Plenty of enterprises—whether they are in the financial space or not—are on the cusp of growth and profitability, but cannot sustain that growth without aligning with regulatory mandates and demonstrating consistency and transparency in data management. Now is the time to take corrective action to ensure the quality and automation necessary to establish a mature data program is in place. While these organizations recognize the need, not all realize that it will be costly to achieve the full efficacy and efficiency associated with quality data. And while it may require some investment up-front, the benefits will quickly offset those costs and lead to greater long-term profitability.
Stay Tuned
In the next installment, we’ll explore—in detail—the regulatory and compliance mandates that add to the urgency of quality and standardization. To learn more, contact AHEAD today.