OPERATIONALIZING EDGE AI: ADAPT NOW OR FAIL WAITING
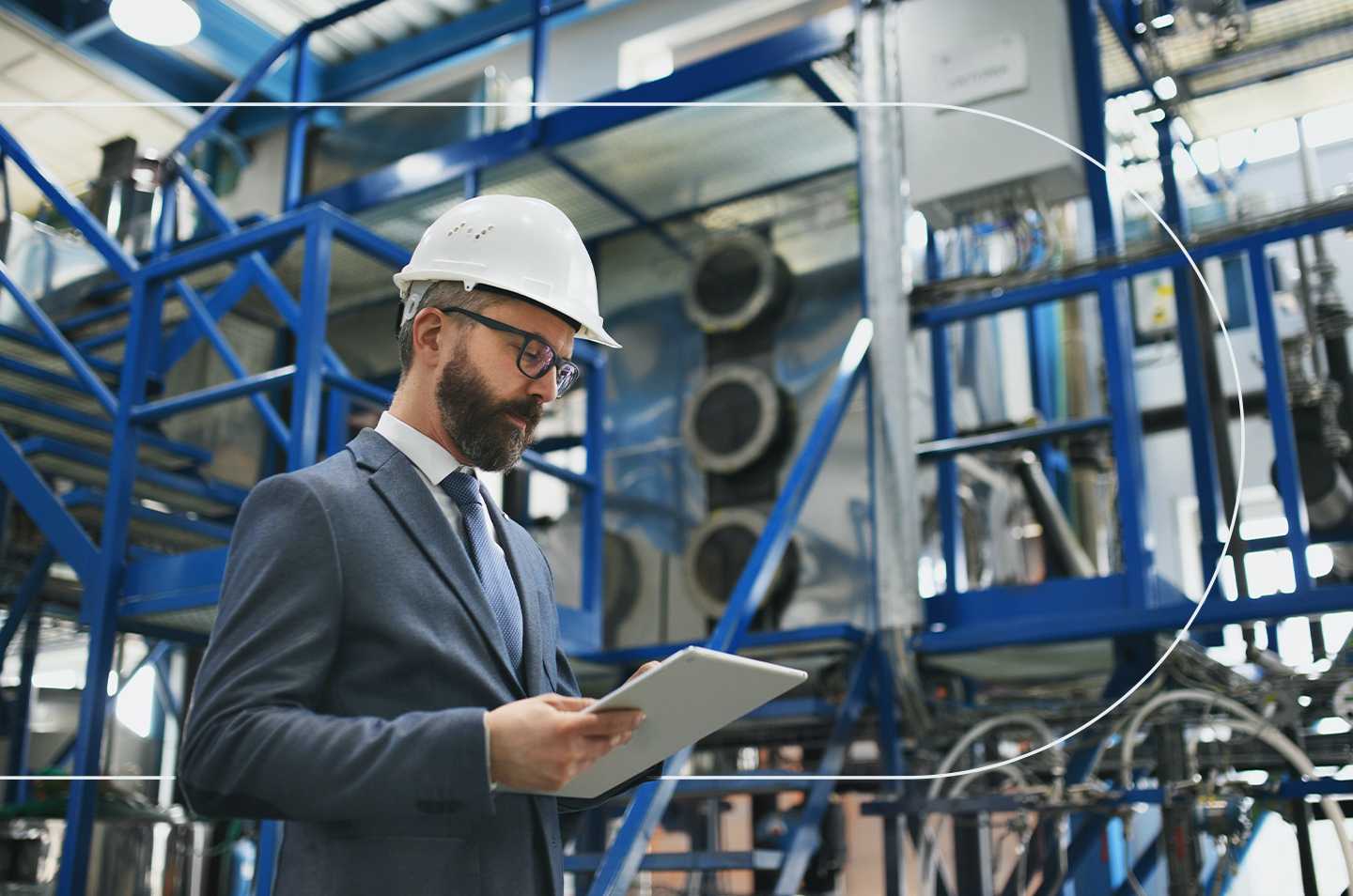
We’re only a few months into 2024, and the AI Gold Rush is in full effect. Computer hardware companies like Supermicro and Dell are cashing in, with share values increasing in percentages by the hundreds. And NVIDIA, the world leader in AI chip manufacturing, has seen its stock boost over 360% in the past 12 months. The message is loud and clear…
If organizing and structuring your data into an operational technology is not at the forefront of your priorities today, prepare to watch your competition leapfrog your innovation tomorrow.
Generative AI has slingshot the world toward overall AI adoption. While not all AI is generative, organizations are seeing the vast capabilities and improvements artificial intelligence can bring. New AI models have driven never-before-seen requirements for inference, especially at the edge. These models are powered by well-architected, GPU-enabled solutions that allow once computationally impossible edge workflows to be processed in near real time. One dominant use case is computer vision, requiring GPU-enabled compute at the edge to make real-time decisions about objects detected in live camera feeds.
It’s no wonder chip makers like NVIDIA, the primary producer of GPU technology, cannot manufacture fast enough for the present demand.
Organizations in industries such as transportation, utilities, manufacturing, retail, and healthcare are using fine-tuned AI models to quickly make decisions against live streams of data coming from an ecosystem of edge sensors and IoT devices. By using real-time AI in daily operations they’re reducing overhead, improving quality and safety, and ultimately driving revenue.
A Deliberate Approach to Operationalizing AI
Consulting with organizations on their AI strategy and roadmap, some of the biggest challenges we need to solve first are:
- Data quality, quantity, and availability
- Governance and compliance
- Data flow
- Model selection
- Infrastructure architecture
- Model tuning and training
When it comes to training or fine tuning an AI model, properly structured data is a foundational starting point. What comes next is aligning the right application frameworks and toolkits with the use case. For example, NVIDIA Metropolis™ vision AI framework helps advance computer vision solutions by providing purpose-built pre-trained models that eliminate the need to build models from scratch.
Once the model is trained, inferencing against real-time data created from sensors like cameras at the edge is when the rubber meets the road. AI inferencing provides real-time insights that automate and accelerate operations.
Deploying Edge AI at Scale
While we’ve established AI and edge work hand in glove to impact an organization’s operational ecosystem, edge computing presents several other challenges, namely managing endpoints at scale:
- Multiple locations and distributed systems at the far edge
- Ongoing health management of devices
- Zero-touch remote management of OS, applications, and firmware
- IT lifecycle management
While these challenges can be overcome, there’s been a void in the industry in terms of automation, obstructing organizations’ ability to orchestrate edge deployments at scale.
New on the scene is edge orchestration software, the missing link for automating edge device management at scale. With the recent release of Dell NativeEdge, for example, organizations now have the ability to centralize edge management and automate provisioning operations across multiple locations, with zero-trust security to protect the applications, data, and users that are running on these endpoints. Zero-touch provisioning simplifies edge operations at scale using automation to manage the edge devices and applications.
Enterprises looking to scale up and scale out their edge AI capabilities can benefit from AHEAD’s AI expertise – from strategy through application, to custom hardware/software integration, to IT lifecycle management. We’re immersed in accelerating the impact of technology and future-proofing IT investments. Waiting to see how AI will be adopted is a posture of the past, with AI becoming the foundation of innovation across all industries, acting now on your AI strategy is a must!
Driving Change in Edge AI
AHEAD is keenly focused on working with enterprises to successfully deploy AI models that will define their future-state in a data-heavy world. Our unique approach is designed to meaningfully improve how edge AI is deployed.
For physical edge devices, we’re able to deliver turnkey solutions at scale versus multiple vendors and touchpoints along the way. We in-source custom manufacturing, integration, kitting, and logistics at our own facilities and see projects all the way through on-site implementation.
Second, we have an advanced approach to managing the lifecycle of these physical assets. We equip clients with Hatch, AHEAD’s proprietary software developed specifically for managing complex IT lifecycles. Hatch consolidates asset lifecycle data from procurement through decommissioning and facilitates device management by synthesizing each asset’s location, associated components, data harvested during configuration, service entitlements, and more.
Finally, we factory-install edge orchestration software onto the devices to achieve plug-and-play simplicity as well as to enable IT teams to remotely manage and update their devices across limitless locations.
AHEAD is a change-maker in the industry, helping clients adapt to rapid adoption of AI and future-proof their organization to be prepared for what’s next.