THE COMPREHENSIVE GUIDE TO BUILDING, ORCHESTRATING, AND MANAGING EDGE AI IMPLEMENTATIONS AT SCALE
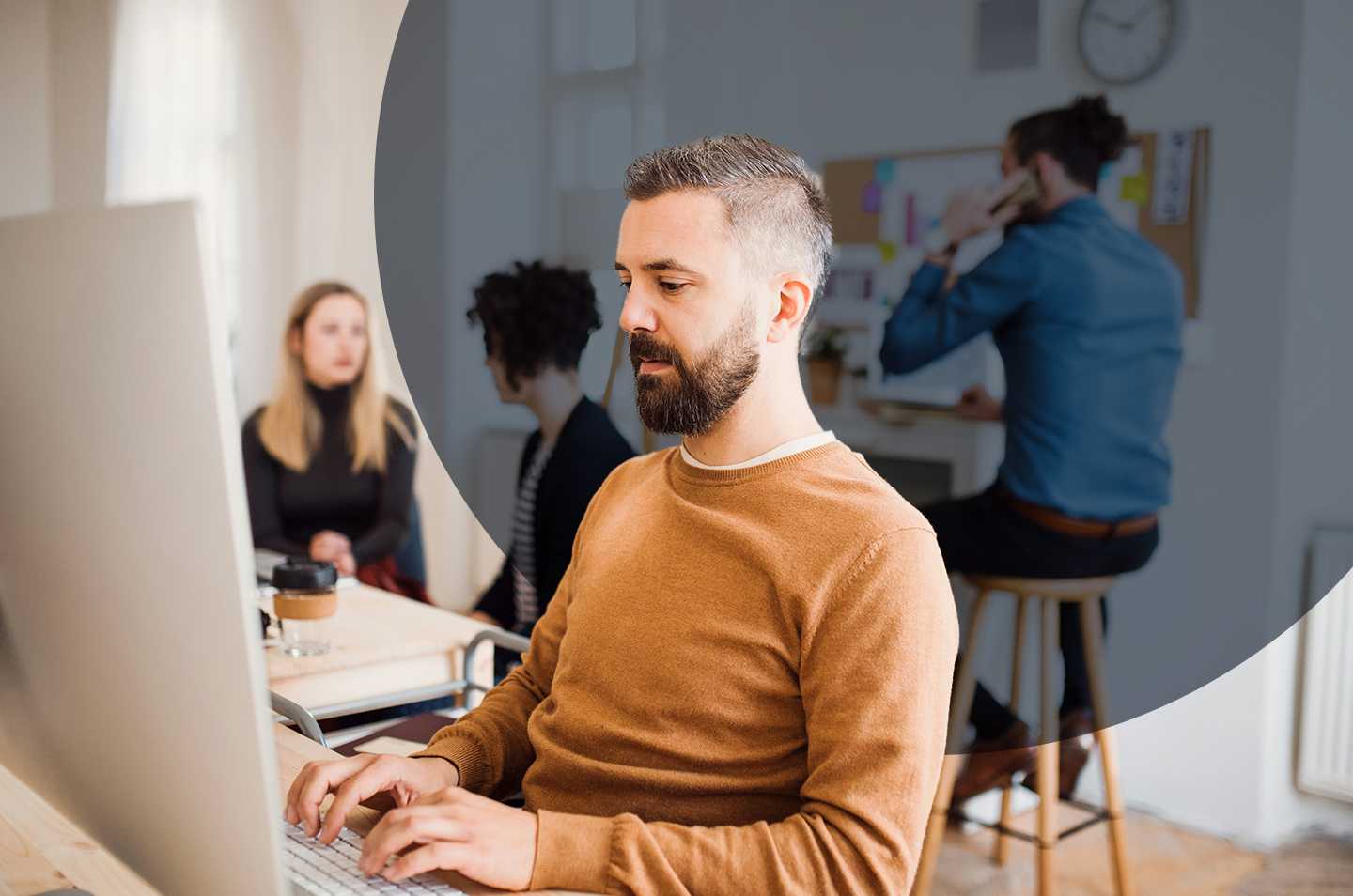
If you’re thinking of investing in next-generations technologies to operationalize edge AI, you’re in good company. In fact, Gartner predicts that “by 2026, at least 50% of edge computing deployments will involve machine learning (ML), compared with 5% in 2022.”
These edge deployments are being used in transportation, utilities, manufacturing, retail, healthcare, and other industries for AI inferencing of sensor data to enable quicker decision making at the edge – resulting in higher efficiency and reduced safety and security risks.
However, there are still many barriers to large-scale edge adoption. Specialty hardware devices can be difficult to design and distribute to far edge locations around the world. There are also lifecycle challenges with managing and orchestrating large edge fleets long after they’ve been deployed.
In this comprehensive (and we mean comprehensive) guide, we’ll discuss the benefits of edge AI solutions and the challenges with implementing them. We’ll also cover ways AHEAD can help build, orchestrate, and manage large-scale edge fleets.